Planned training:
Soon!
Price:
4140€
(When paying the full amount, a 10% discount is applied immediately)
The course is comprehensive, aimed at both beginners and those with basic knowledge who want to deepen their understanding of modern technologies of artificial intelligence and data analysis...
What after training?
The principle of mentoring after the training - throughout the month, if you have any questions, you can contact the school's lecturer. Also, you will be able to use the school's classrooms, both during and after the training. The principle of mentoring after the training - throughout the month, if you have any questions, you can contact the school lecturer. Also, you will be able to use the school classrooms, both during and after training.
Training program
1. Introduction to Python
- Getting to know the class
- Python history, installation on your computer, examples
- The first Hello World Python program, Python programming philosophy
2. Code versioning
- Basics of code versioning
- Git and GitHub
- Git installation and environment preparation, practice
3. Python basics
- int, float, boolean and mathematical operations
- string, their methods, formatting, slice
- if conditions, input(), range(), for loop
- list, tuple, set
- dictionary
- whileloop
- functions, None, lambda, recursion, local vs. global scope
- error types, management and their generation try/except, raise
- Intermediate knowledge test
- Working with documents, os and pathlib libraries, relative vs. absolute path, .txt, .json, .yaml formats, pickle library
- Managing Python packages/libraries, importing modules, Python virtual environments, generating .exe from a .py script
4. Python object-oriented programming
- Definition of classes and OOP, examples
- Class declaration, constructor, destructor, creation of class units/objects (instance).
- Class methods and their calls
- Class inheritance, advantages and disadvantages, polymorphism, creating an abstract class
- designing class structures and methods
- Use of object-oriented programming in data analysis, real examples
- Class static properties and methods
5. Python data analytics
- Principles of web scraping, BeautifulSoup and other libraries, legal restrictions and ethical issues
- Analysis of textual data, Regex
- Numpy library, working with multidimensional data, data masks
- DataTables, Pandas library, Series and DaraFrame
- Data visualization, Matplotlib, Seaborn libraries. Interactive graphs with the plotly library
- Visual data analysis and processing. OpenCV, Pillow libraries
6. Python work with databases
- What is a database, SQL vs. noSQL, examples
- Installing a MySQL or PostgreSQL database on your computer, practice with the application's UI
- Reading data from the database.
- Creating and editing tables, saving and deleting data in the SQL database
- Extracting data from a database with Python
- Data Transformation, Management and Analysis with SQL and Python (Pandas Library)
1. Theory of Machine Learning
- What is Machine Learning (ML), AI vs. ML, how it differs from traditional programming, ML project flow
- History of Machine Learning
- Types of learning (supervised, unsupervised/self-supervised, reinforcement) and examples of use
- Structured (categories, numbers, etc.) vs. unstructured data (text, sound, etc.)
2. Supervised learning
- Supervised learning and usage examples
- Classification problem, training data split (train-test split), model evaluation metrics
- Scikit-learn library. Linear models, K-Nearest Neighbors, Decision Trees, Bagging and Boosting methods. underfit vs. overfit
- Binary vs. Multiclass vs. Multi-label classification. Logistic function
- Regression, regression model estimation metrics
- Practice with regression models (Scikit-learn library)
- Non-negative regression and example situations. ReLu function, Poisson and Tweedie data distributions
3. Unsupervised
- Unsupervised (self-supervised) learning and usage examples
- Data clustering, what is good clustering?
4. Feature engineering
- Data normalization, data encoding and why it is important
- Feature selection and Dimensionality reduction. Principal Component Analysis
- Model hyperparameter tuning (hyperparameter tuning), cross-validation
5. Evolutionary algorithms
- Theory and terminology of evolutionary algorithms
- Practice with Evolutionary Algorithms. Python DEAP library
You need a computer with an NVidia GPU with a Compute Capability of at least 6.0
or use Google Colab with GPU (free version doesn't always work due to busyness)
1. Theory of artificial neural networks (ANN).
- History of artificial neural networks, examples of use, what is an artificial neuron, activation functions
- Structure of artificial neural network (Multilayer Perceptron), foward propogation, backpropogation
- Loss/cost function, its representation as a mountainous surface, Gradient descent
- Learning cycle
2. ANN Practice with Pytorch
- Introducing and recording Pytorch
- Building an Artificial Neural Network with Pytorch to Classify Fashion-MNIST Photos. Model training, evaluation of results
- Loss curve, early stopping, how useful is Dropout
3. Convolutional Neural Networks (CNN)
- What is a photo for a computer? What problems do you have when trying to understand what is shown in the picture
- Filters (image kernel), convolutional network structure, pooling layer
- Building a CNN with Pytorch to classify photos (eg CIFAR10). Model training, evaluation of results
- Data augmentation and its impact on training
- Transfer Learning. How to adapt trained models to your needs
- Object detection in photos. YOLO, MobileNet models. Object recognition model evaluation metrics
- Semantic segmentation vs. instance segmentation. Mask R-CNN, Segment Anything (SAM), YOLACT models
4. Recurrent Neural Networks (RNN)
- Data streams (audio, video, text) and looking back
- Definition, uses and limitations of RNN network
- Music generation with RNNs
5. Generative Adversarial Networks (GANs)
- GAN definition, usage examples
- Training a GAN model to restyle a photo into a Van Gogh style
6. Transformer neural network (Transformer)
- Transformer definition, how data importance (attention) is calculated, usage examples, what are the disadvantages of this model
- Training a Transformer model for photo classification
1. NLP
- NLP Basics, History and Uses
- TF-IDF, bag of words, Spacy library
- Word embeddings, their types, pluses and minuses
- Text classification
- Transformer networks of text. Hugging face platform and library
- Text generation with Transformer networks
1. Reinforcement learning
- What is Reinforcement learning, history, usage examples, reward function, Exploration vs. Exploitation, action selection
- Reinforcement learning model training in one of the Gymnasium environments
1. Task solutions and consultations
- Solutions for tasks to be solved throughout the course, consultations for the final project
1. Final project presentations
- Final project presentations
1. Communication skills
- Active listening
- Effective communication
- Dealing with difficult customers
- Creating a connection
2. Problem solving strategies
- Problem analysis and diagnostics
- Determination of solutions
- Troubleshooting methods
- Root cause analysis of the problem
- Latest problem solving technologies
- How to get feedback from managers
- Professional development
3. Time management and organization
- Prioritization of tasks
- Workload management
- Delegation and collaboration
4. Emotional intelligence
- Understanding and managing emotions
- Empathy and compassion
- Conflict resolution
- Stress management
5. How to learn more and faster and less
to forget
- 5 minute brain exercise
- Clear your "mental fog" with these 5
delicious brain foods - Learn a powerful memory technique to
you would never miss a meeting
- The operation of artificial intelligence. How is it different from a human?
- Creating a request (Prompt). What is the difference between a good query and a bad one?
- Validation of results. Checking the received data for suitability in a specific case
- Versioning Policy. Identification of the used software (vendor) version in the request
- Artificial intelligence plugins in the code editor (IDE). Advantages and disadvantages
- Generating comments using artificial intelligence
- Creating tests with the help of a plugin
- Code optimization with the help of a plugin
Vilnius coding School CERTIFICATE
strong evidence that you are ready career ITEM!
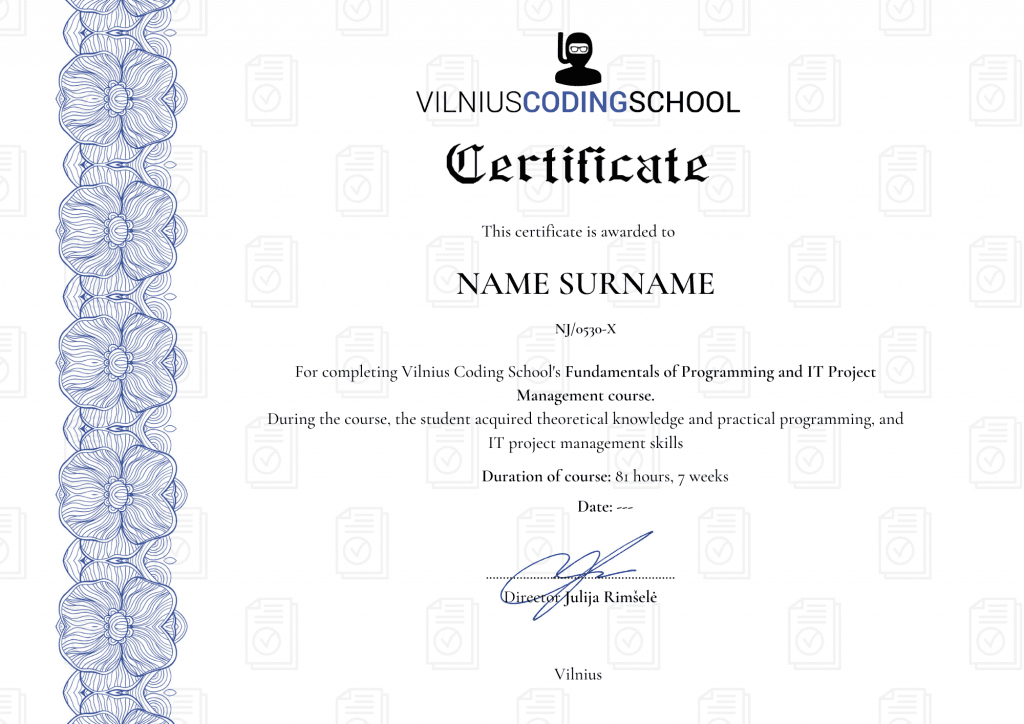
Official certificate approved by the Education and Science Register
Recognized by the strongest Lithuanian and international companies
It proves that you have studied with the best specialists in your field
Reliable proof of acquired skills
Certificate issued by a company developing transparent activities in Lithuania
This certificate confirms that you attended at least 95% of the course lectures in real time with the lecturer, completed and defended the final thesis
Discount system
Normal cost of training
4140 €
BY PAYING THE FULL AMOUNT IMMEDIATELY
we apply a 10% discount!
€ 3726
(Full price 4140 €)
Applies if you pay the full amount at the time of pre-registration
ALUMNI
we apply a 15% discount!
€ 3519
(Full price
4140 €)
Applies to all school graduates for all training and weekend workshops
RECOMMEND US TO A FRIEND
we apply a 10% discount!
€ 3726
(Full price
4140 €)
Applies if you come to study as a couple